All are welcome at the Symposium on Geometry Processing 2024.
Attendees, presenters, volunteers and sponsors are subject to the SGP
2024 Code of Conduct.
Keynotes
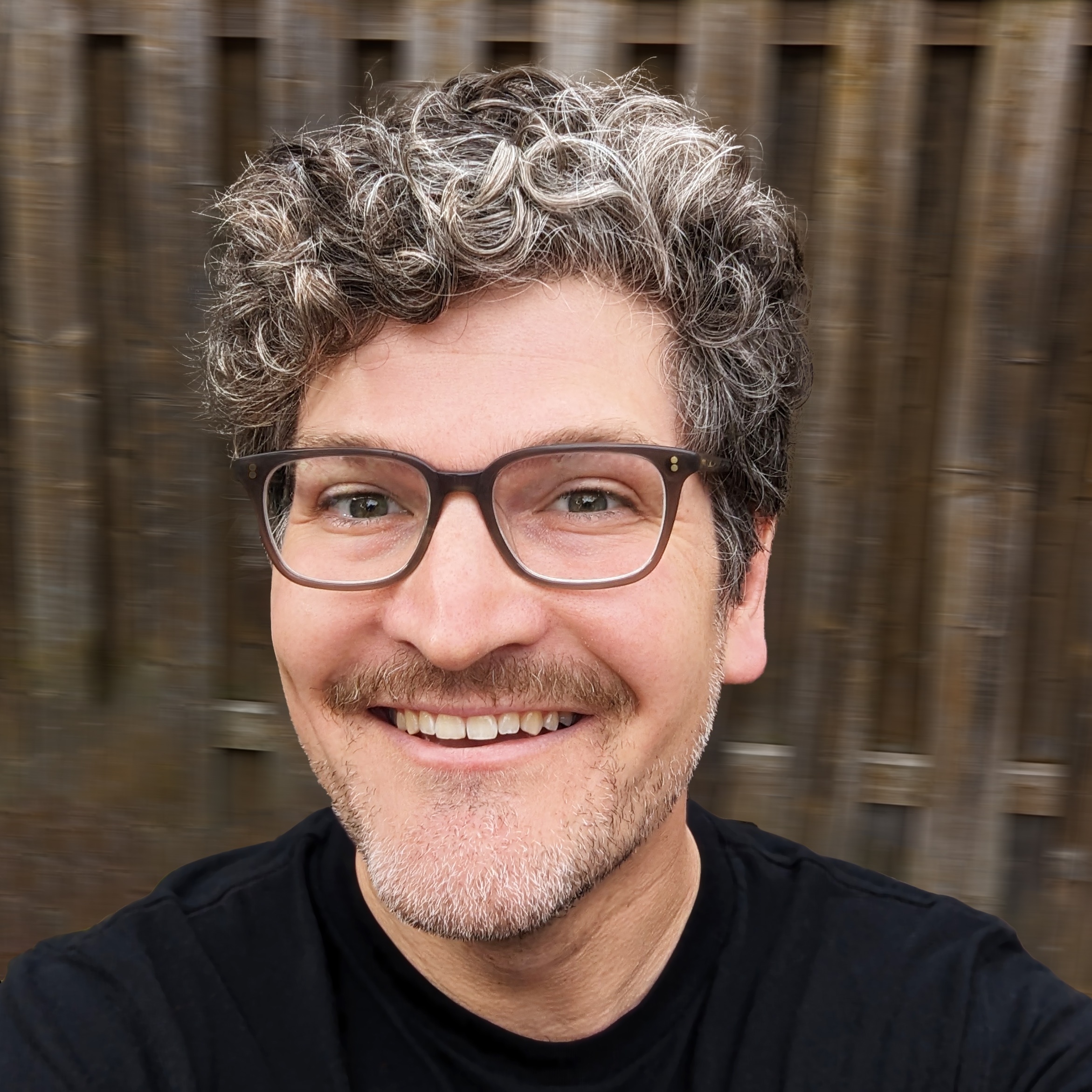
Alec Jacobson
University of Toronto & Adobe Research
The Triangle is Dead, Long Live the Triangle!
The geometry processing community has made tremendous progress working with discrete surfaces represented as explicit meshes of triangles. However, recent advances in computer vision and machine learning have demonstrated the power & seeming superiority of unstructured implicit representations. In this talk, I will present thoughts on how our research has been too attached to the triangle, how we can benefit from embracing alternative representations, and why ultimately triangle meshes still have an important role.
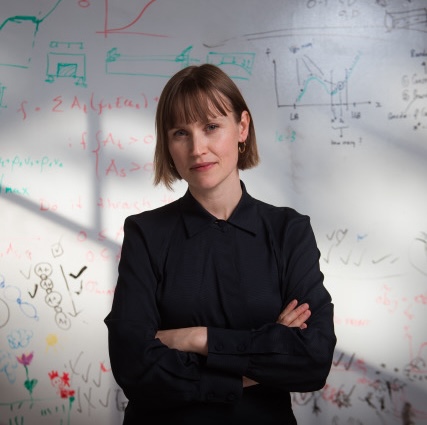
Josephine Carstensen
Massachusetts Institute of Technology
Human-guided geometry processing in high-performance engineering design
Topology optimization has gained traction as a design method for high-performing engineering components and structures. It is a computational approach that generates efficient material layouts, tailored to a user’s specific design requirements. To take full advantage of its exploratory power, topology optimization leaves the user as a passive observer who initiates the design process and assesses the quality of the design upon completion. The resulting engineering designs are typically high-performing and have high levels of geometric complexity. However, ensuring the physical performance is adequately predicted by a fully automated design approach requires the inclusion of all relevant operating conditions, mechanical behaviors, and fabrication constraints. This requirement limits the widespread use of topology optimization. This talk will focus on recent contributions that address this barrier by introducing human-guided geometry processing as designs are generated. The human experience is actively leveraged to interactively alter local geometric feature size requirements or to encourage similarity to drawn sketches. Integrating human-guided geometry processing is shown to improve known and complex performance considerations related to both mechanical behavior and manufacturability of the designs.
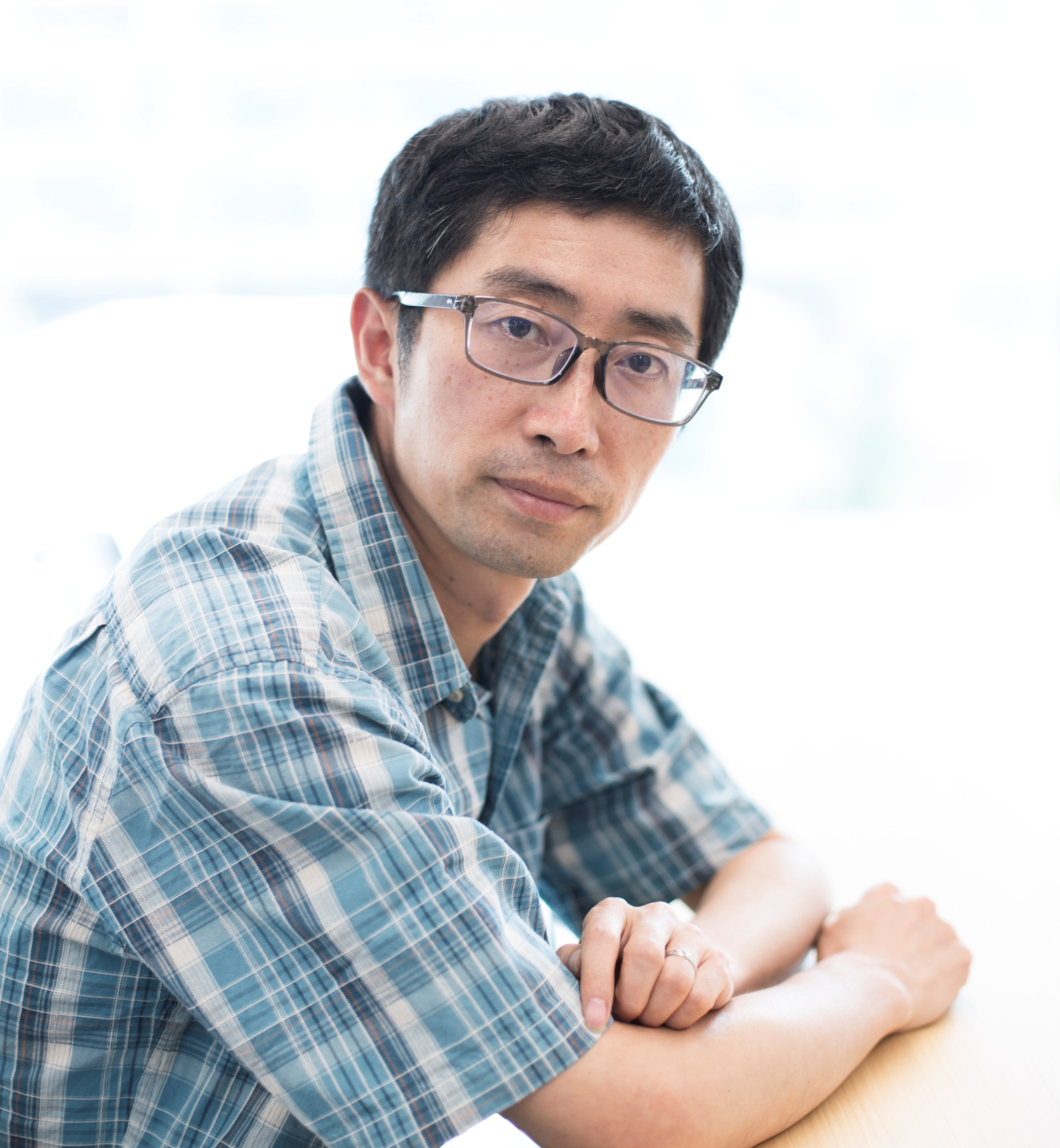
Xin Tong
Microsoft Research Asia
Deep Learning based 3D Understanding and Generation
Over the past decade, the advancement of deep learning techniques has profoundly transformed the fields of computer vision and natural language processing. However, the distinct characteristics of 3D geometric data present obstacles to the application of deep learning in 3D understanding and generation tasks. In this talk, I will first explore the technical challenges encountered in 3D deep learning. Subsequently, I will present our research work aimed at addressing these challenges in deep learning based 3D understanding and generation. Finally, I will discuss the prospects, highlighting the potential opportunities and challenges in the field of 3D deep learning.